About Author
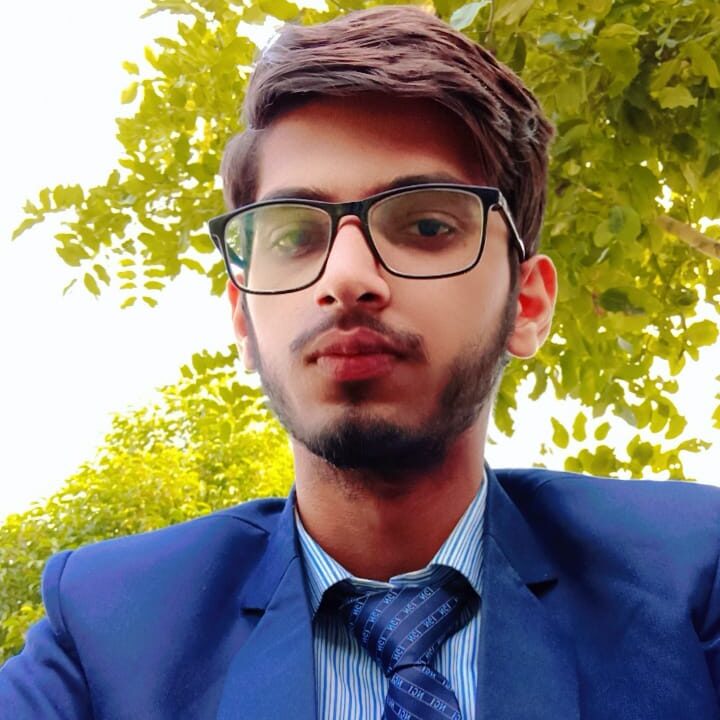
Sudhanshu Gour
Hi, I’m Sudhanshu Gour, a specialist in Digital Marketing. I excel in SEO, content marketing, and social media. I’m passionate about staying ahead of trends and sharing knowledge through Writing Blog Post.
Hi, I’m Sudhanshu Gour, a specialist in Digital Marketing. I excel in SEO, content marketing, and social media. I’m passionate about staying ahead of trends and sharing knowledge through Writing Blog Post.
Leave a Reply